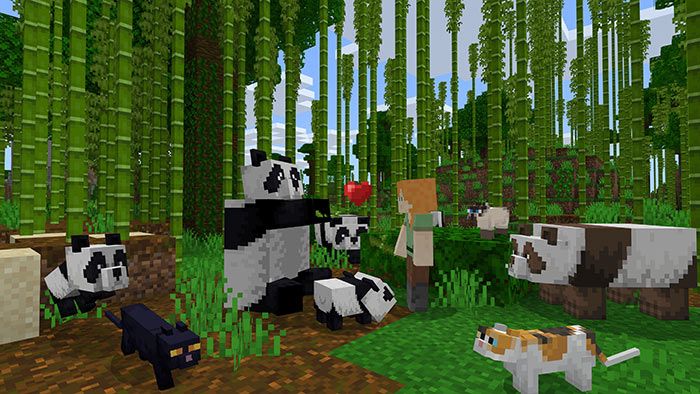
Minecraft as AI Benchmark: A Creative Approach to Model Evaluation
Traditional AI benchmarking methods are struggling to keep pace with the rapid advancements in generative AI. As a result, developers are exploring innovative approaches to evaluate the capabilities of these models. One such approach involves using Minecraft, the popular sandbox-building game owned by Microsoft.
MC-Bench: AI Models Face Off in Minecraft
The Minecraft Benchmark (MC-Bench) website is a collaborative platform where AI models compete in creating Minecraft builds based on given prompts. Users can vote on which model performs better, revealing the AI behind each creation only after casting their vote.
Adi Singh, a high school student and the creator of MC-Bench, highlights the familiarity of Minecraft as its key advantage. As the best-selling video game of all time, even those unfamiliar with the game can easily assess the quality of a blocky pineapple representation.
“Minecraft allows people to see the progress [of AI development] much more easily,” Singh told TechCrunch. “People are used to Minecraft, used to the look and the vibe.”
MC-Bench currently has eight volunteer contributors. Companies like Anthropic, Google, OpenAI, and Alibaba support the project by subsidizing the use of their products for running benchmark prompts. However, they have no other affiliation with the project.
Singh envisions expanding MC-Bench beyond simple builds to incorporate more complex, goal-oriented tasks. He believes that games offer a safer and more controllable environment for testing agentic reasoning compared to real-life scenarios.
“Games might just be a medium to test agentic reasoning that is safer than in real life and more controllable for testing purposes, making it more ideal in my eyes.” Singh said.
The Challenges of Traditional AI Benchmarks
Other games like Pokémon Red, Street Fighter, and Pictionary have also been used as experimental AI benchmarks, highlighting the difficulties in traditional AI benchmarking.
Standardized evaluations often provide AI models with an unfair advantage due to their training. Models excel at specific, narrow problem-solving tasks, particularly those involving rote memorization or basic extrapolation.
For example, GPT-4's high score on the LSAT contrasts sharply with its inability to count the number of "R"s in "strawberry." Similarly, Claude 3.7 Sonnet's strong performance on a software engineering benchmark is undermined by its poor performance in playing Pokémon.
MC-Bench: A More Accessible Benchmark
MC-Bench functions as a programming benchmark, requiring models to write code to create builds based on prompts like "Frosty the Snowman" or "a charming tropical beach hut."
However, the visual nature of Minecraft makes it easier for users to evaluate the quality of a build compared to analyzing code. This broader appeal allows MC-Bench to gather more data on which models consistently perform better.
The correlation between MC-Bench scores and real-world AI usefulness remains a topic of discussion. However, Singh believes that the scores provide valuable insights.
“The current leaderboard reflects quite closely to my own experience of using these models, which is unlike a lot of pure text benchmarks,” Singh said. “Maybe [MC-Bench] could be useful to companies to know if they’re heading in the right direction.”
2 Images of AI Benchmarking Minecraft:
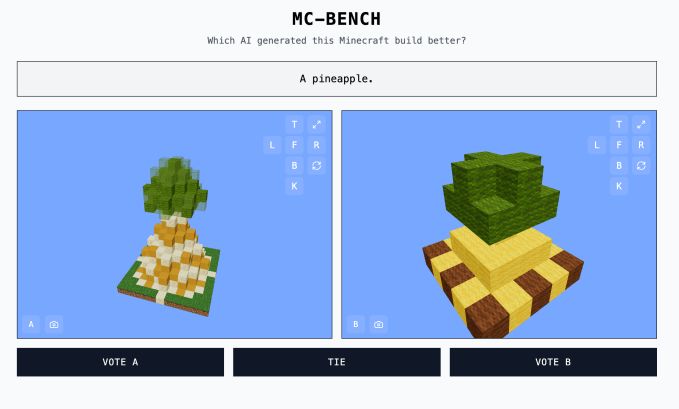
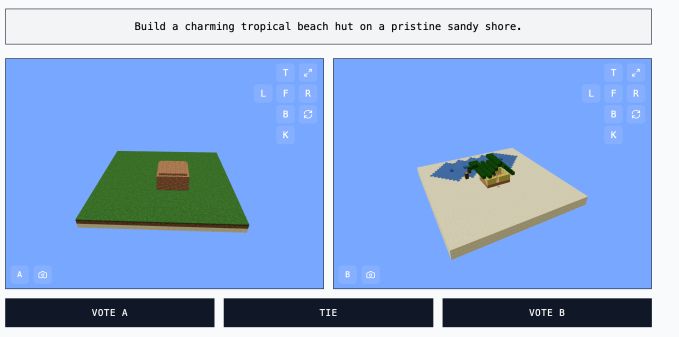
Source: TechCrunch